티스토리 뷰
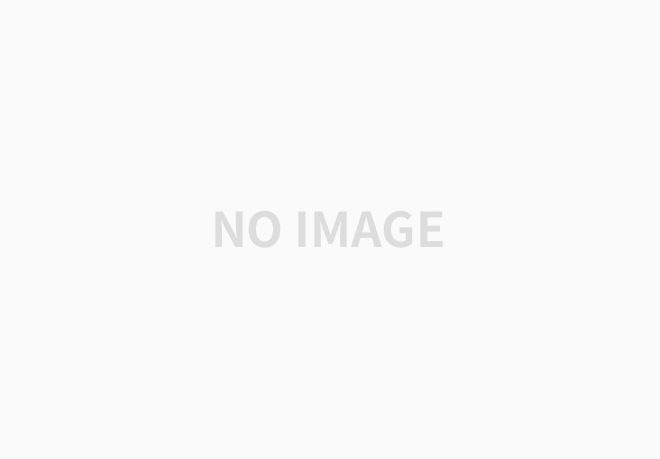
모듈화된 모델을 사용하고 있는데, 하나의 모듈을 pretrain 시킨 후 전체 모델에 통합시킨 후 다시 학습시켰을 때 성능이 떨어지는 현상이 있었다. Formulation이 아래와 같을 때
Formulation: input -> middle output -> final output
input->middle output을 pretrain했다는 것이고, 이 때 middle output의 error가 50이었는데, 전체 모델에 통합시킨 후 다시 학습시켰을 때 middle output의 error가 55로 안 좋아졌다는 말이다.
원인은 2가지가 있는데,
1. pretrain했을 때의 learning rate와 전체 모델에 통합시킨 후에 학습시킬 때의 learning rate가 다르다는 점,
2. pytorch batch normalization이 evaluation 때 default로 축적된 running mean/variance를 쓴다는 점.
2번이 이 글의 메인이다. 1번은 간단하게 말해서 input->middle output의 decay된 최종 learning rate가 1e-5인데, 다시 전체에서 학습시킬 때는 learning rate가 1e-3이면 문제가 생길 수 있다는 것이다. 전체에서는 middle output->final output을 처음 학습시키는거니까 전체 learning rate로 1e-3으로 초기화했었다. 다시 생각해보니 learning rate를 모듈별로 다르게 주면 이 문제는 해소될 수 있겠다.
본론으로 돌아와서, pytorch batch normalization은 원래의 batch norm 정의와 다르게 evaluation time 때 input으로 들어온 data의 mean, variance를 쓰지 않고
따라서 pretrain을 한 후 전체 모델에서 추가로 조금이라도 학습시킨다면 이 running mean/variance가 update rule에 따라 변하기 때문에 test time때 다른 middle output이 나올 수 있다
the update rule for running statistics here is \hat{x}_\text{new} = (1 - \text{momentum}) \times \hat{x} + \text{momentum} \times x_t , where \hat{x} is the estimated statistic and x_t is the new observed value.
'Research (연구 관련)' 카테고리의 다른 글
transductive learning VS inductive learning (0) | 2020.04.07 |
---|---|
C++ reference variable (0) | 2020.04.02 |
install nvidia-driver & hangul in Ubuntu18.04 (0) | 2020.01.23 |
최적화와 머신러닝 (Optimization and ML) (0) | 2019.11.15 |
Virtual Camera / Left handed rule (0) | 2019.09.12 |
- Total
- Today
- Yesterday
- Pose2Mesh
- 인터뷰
- Virtual Camera
- 에디톨로지
- densepose
- 머신러닝
- spin
- 헬스
- world coordinate
- nohup
- Generative model
- pyrender
- focal length
- 2d pose
- deep learning
- 컴퓨터비전
- pytorch
- VAE
- camera coordinate
- Docker
- 비전
- 피트니스
- Transformation
- Machine Learning
- 컴퓨터비젼
- demo
- 문경식
- part segmentation
- Interview
- nerf
일 | 월 | 화 | 수 | 목 | 금 | 토 |
---|---|---|---|---|---|---|
1 | 2 | 3 | 4 | 5 | ||
6 | 7 | 8 | 9 | 10 | 11 | 12 |
13 | 14 | 15 | 16 | 17 | 18 | 19 |
20 | 21 | 22 | 23 | 24 | 25 | 26 |
27 | 28 | 29 | 30 | 31 |