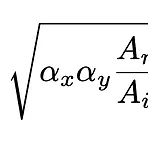
2019 ICCV 논문으로 연구실 선배인 경식이형의 논문이다. 이 포스트의 목적은 이 논문의 모듈 중 하나인 'RootNet'에 대해서, 그 중에서도 논문 자체의 contribution보다는, focal length, camera-object distance (depth), per-pixel distance factor에 대해 다룬 내용을 이해하는 것이다. RootNet의 output은 human root joint의 coordinate인 (x,y,Z)이다. x,y는 img coordinate이고, Z는 camera-centered coordinate system 상의 coordiante으로 root joint의 depth다. inference time 때 x,y는 Z를 구한 후에 Z값과 미리 가정한 f..
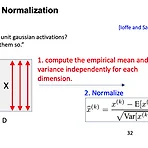
모듈화된 모델을 사용하고 있는데, 하나의 모듈을 pretrain 시킨 후 전체 모델에 통합시킨 후 다시 학습시켰을 때 성능이 떨어지는 현상이 있었다. Formulation이 아래와 같을 때 Formulation: input -> middle output -> final output input->middle output을 pretrain했다는 것이고, 이 때 middle output의 error가 50이었는데, 전체 모델에 통합시킨 후 다시 학습시켰을 때 middle output의 error가 55로 안 좋아졌다는 말이다. 원인은 2가지가 있는데, 1. pretrain했을 때의 learning rate와 전체 모델에 통합시킨 후에 학습시킬 때의 learning rate가 다르다는 점, 2. pytorch ..
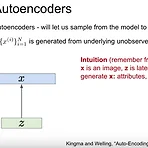
이전 포스트에서 왜 Generative Models의 정의가 P(X,Y)를 구하는 게 아니라 왜 P(X)를 구한 후 샘플링하는 것이 목적이라고 하는 지 이해가 안 간다고 하였다. 여러 VAE를 설명하는 해외/국내 블로그들이 시작할 때 P(X)가 목표다 라고 하는데, 실제로는 Given Y에 대해 가장 그럴듯한 데이터 X를 생성하는 것이 목표이므로 P(X,Y)가 목표라고 생각한다. 곰곰이 생각해본 결과, 결과적으로 Generative Models 중 하나인 VAE가 결국 P(Y|X), P(X,Y), P(X|Y)를 모두 학습하기 때문에 P(X) / 데이터의 진정한 분포를 학습한다고도 할 수 있는 것 같다. GAN이 Implicit하게 P(X)를 구한다는 표현은 P(Y|X), P(X,Y), P(X|Y)를 따로..
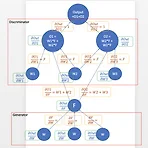
GAN을 공부하다가 tutorial 코드에 netD(fake.detach())가 어떤 원리인 지 이해가 안 갔다. 의도야 설명에 나온대로 netG에 backpropagation이 안되도록, 즉 첫번째 스텝에서는 netD만 학습하려는 것이라는 건 알겠다. (code: pytorch gan tutorial) ## Train with all-fake batch # Generate batch of latent vectors noise = torch.randn(b_size, nz, 1, 1, device=device) # Generate fake image batch with G fake = netG(noise) label.fill_(fake_label) # Classify all fake batch with D..
- Total
- Today
- Yesterday
- Interview
- Docker
- pyrender
- 헬스
- camera coordinate
- pytorch
- Generative model
- part segmentation
- 인터뷰
- 2d pose
- nerf
- Virtual Camera
- deep learning
- 컴퓨터비전
- 컴퓨터비젼
- VAE
- 머신러닝
- demo
- spin
- Pose2Mesh
- Transformation
- 피트니스
- nohup
- world coordinate
- focal length
- 비전
- 에디톨로지
- densepose
- 문경식
- Machine Learning
일 | 월 | 화 | 수 | 목 | 금 | 토 |
---|---|---|---|---|---|---|
1 | ||||||
2 | 3 | 4 | 5 | 6 | 7 | 8 |
9 | 10 | 11 | 12 | 13 | 14 | 15 |
16 | 17 | 18 | 19 | 20 | 21 | 22 |
23 | 24 | 25 | 26 | 27 | 28 |