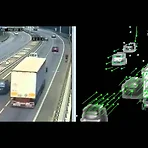
This post explains my understanding on Aperture Problem in Optical Flow and Lukas-Kanade method which is one of approaches to solve the problem. First, Optical Flow is a one way to estimate motion flow by analyzing pixel images of continous frames. Assuming Bright Constancy which leads to Gradient Constancy, one can define a line in the (u, v) space where u, v stand for dx / dt , dy / dt. But we..
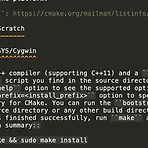
cmake는 os에 상관없이 make를 수행할 수 있는 meta make이다. UNIX 중심 make에 대한 대안이라나. make자체는 프로그램 빌딩 도구로 위키에 다음과 같이 정의되어 있다. make는 소프트웨어 개발을 위해 유닉스 계열 운영 체제에서 주로 사용되는 프로그램 빌드 도구이다. 여러 파일들끼리의 의존성과 각 파일에 필요한 명령을 정의함으로써 프로그램을 컴파일할 수 있으며 최종 프로그램을 만들 수 있는 과정을 서술할 수 있는 표준적인 문법을 가지고 있다.위의 구조로 기술된 파일(주로 Makefile이라는 파일명)을 make가 해석하여 프로그램 빌드를 수행하게 된다. https://cmake.org/download/ 여기서 자기 OS(난 MACOS)에 맞는 install package를 다운로..
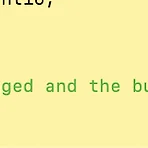
credit to https://catonmat.net/sudo-vim How to save read-only files in vim How many times have you had a situation when you open a file for editing, make a bunch of changes, and discover that you don't have the rights to write the file? This has happened to me a lot. It usually goes like this. You open a file and you forget to us catonmat.net This is a merely Korean translation for the post abov..
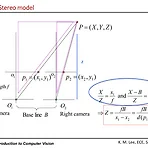
Wikipedia의 영어설명은 사람 눈의 관점에 쓰여있고 Visualization도 눈으로 되어 있따. Disparity의 단위가 Angle이라 되어있어서 읭 싶었다. 한글 설명을 찾으려다 그냥 수업 교재에 잘 설명되어 있었다. d(p1) = x1 - x2 이 바로 Binocular Disparity이고, 두 Stereo Images에서 대응되는 두 점 사이의 distance이다. 말 그래도 거리라기보단 차이! 각각의 이미지에서 두 점이 갖는 픽셀위치를 계산해서 빼주면 된다.
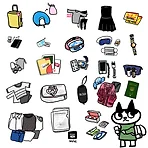
Ad hoc: "이것을 위해" 즉 "특별한 목적을 위해서" == "for this purpose" Object Categorization (Bags of Words Model) 수업 중 Ad hoc Category가 잠깐 언급됐다. 일반적인 카테고리들(Common Categories)로는 과일, 바지 등이 있고 그 외의 카테고리들로는 functional categories, ad hoc categories가 있으며 각각 chairs = “something you can sit on”, “something you can find in an office environment”가 예시로 들어졌는데 둘이 무슨 차이인지, 특히 Ad-hoc category 자체의 정의가 무엇인지 헷갈렸다. 그래서 구글링을 엄청했는..
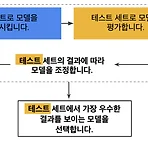
머신러닝에서 Validation Set은 왜 필요한가? Train Set, Test Set 둘로만 나눠서 Test set 결과만 가지고 모델들을 비교하면 안되나? 표현을 좀 바꿔보자. 첫번째 사진에서 Test Set을 Validation Set이라고 하고, 왜 Validation Set 결과만 가지고 모델들을 비교하면 안될까? 왜 Test Set이 필요할까? 일단 Validation Set은 모델을 학습시킬 때 직접적으로 사용되지 않지만, Validation Set에서 우수한 결과를 내기 위해 사람이 Validation Set 결과를 보고 계속 Hyperparameter Tuning을 한다. Validation Set에서 최우수한 결과를 내기 위해Hyperparameter들을 fitting한다고도 할 수..
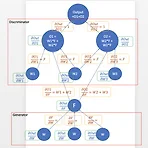
GAN을 공부하다가 tutorial 코드에 netD(fake.detach())가 어떤 원리인 지 이해가 안 갔다. 의도야 설명에 나온대로 netG에 backpropagation이 안되도록, 즉 첫번째 스텝에서는 netD만 학습하려는 것이라는 건 알겠다. (code: pytorch gan tutorial) ## Train with all-fake batch # Generate batch of latent vectors noise = torch.randn(b_size, nz, 1, 1, device=device) # Generate fake image batch with G fake = netG(noise) label.fill_(fake_label) # Classify all fake batch with D..
https://zzsza.github.io/data/2018/06/25/upsampling-with-transposed-convolution/ Up-sampling with Transposed Convolution 번역 Naoki Shubuya님의 Up-sampling with Transposed Convolution을 허락받고 번역한 글입니다. 번역이 어색한 경우엔 영어 표현을 그대로 사용했으며, 의역이 존재할 수 있습니다. 피드백 언제나 환영합니다! zzsza.github.io 한번 더 이해해서 내 단언로 정리해보자
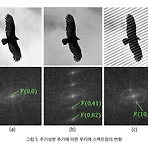
아직도 다 깊이 이해하지는 못하지만 (증명이라던가) 수식적으로 magnitude 공간(=스펙트럼 이미지)와 원본 이미지가 어떻게 대응되는 지 이해 Phase가 magnitude보다 중요한 정보라는 사실에 대한 직관적 이해 위 2가지를 다크프로그래머님의 글을 읽고 얻을 수 있었다. 수식적으로 magnitude 공간에서 peak가 원점에서 멀어질 수록 주파수가 크고, 원본 이미지의 주기성분의 주기는 작다. 또한 u, v는 각각 x, y에 대응되기 때문에(F(u, v)는 x축 방향으로 주파수(frequency) u, y축 방향으로 v인 주기함수 성분의 계수) magnitude공간에서의 peak의 나열방향과 원본 이미지에서의 주기성분의 방향이 일치한다. Phase가 magnitude보다 중요한 정보라는 사실에 ..
- Total
- Today
- Yesterday
- 에디톨로지
- Docker
- 2d pose
- 인터뷰
- 헬스
- VAE
- pyrender
- 컴퓨터비전
- 비전
- 문경식
- 머신러닝
- 컴퓨터비젼
- world coordinate
- nerf
- densepose
- Pose2Mesh
- nohup
- Transformation
- demo
- spin
- focal length
- camera coordinate
- 피트니스
- Machine Learning
- Generative model
- pytorch
- deep learning
- Virtual Camera
- part segmentation
- Interview
일 | 월 | 화 | 수 | 목 | 금 | 토 |
---|---|---|---|---|---|---|
1 | ||||||
2 | 3 | 4 | 5 | 6 | 7 | 8 |
9 | 10 | 11 | 12 | 13 | 14 | 15 |
16 | 17 | 18 | 19 | 20 | 21 | 22 |
23 | 24 | 25 | 26 | 27 | 28 |